 |
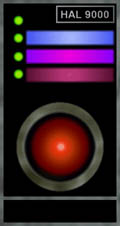 |
Seminar-style Graduate Course on advanced topice in machine learning and
statistical modeling.
Instructor: Yann LeCun, 715 Broadway, Room 1220, x83283, yann [ a t ] cs.nyu.edu
Classes: Wednesdays 2:00-3:50PM, Room 1221, 719 Broadway.
Office Hours for Prof. LeCun: by appointment
Click here for schedule and course material >>>
|
The course will be a combination of seminar-style sessions reviewing
key papers from the literature and tutorial lectures on advanced
research topics. The topics covered in the course will include:
|
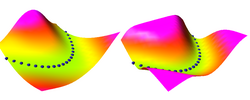 Energy-Based Models
|
- advanced topics in graphical models, approximate inference.
- variational methods for inference and learning
- unsupervised learning, dimensionality reduction,
and independent component analysis
- advanced sampling methods for inference and learning
(Hybrid Monte-Carlo, Contrastive Divergence)
- Markov Random Fields and Conditional Random Fields
- Energy-based models.
- Advanced discriminative methods for sequence labeling.
- Advanced topics in reinforcement learning and
Markov decision processes.
Who Can Take This Course? |
This course is primarily intended for PhD students whose research work
is related to machine learning, and for advanced MSc students who
intend to do thesis work on machine learning.
Prerequisite: some background in machine learning.
Having taken either "Machine Learning and Pattern Recognition"
or "Foundations of Machine Learning" is recommended, but
not an absolute requirement. If you have not taken one of the
above course, you must obtain authorization from the instructor
before registering for credit. If you are one of the few lucky
undergrads who have passed the MLPR class and want to take
thins class, send email to the instructor.
Everyone is welcome to audit the classes.
|
|
This course can be useful to all students who need to develop
new statistical modeling methods. This includes students in CS
(AI, Vision, Graphics), Math (System Modeling), Neuroscience (Computational
Neuroscience, Brain Imaging), Finance (Financial modeling and
prediction), Psychology (Vision), Linguistics, Biology (Computational
Biology, Genomics, Bio-informatics), and Medicine (Bio-Statistics,
Epidemiology).
The topics studied in the course will include two types of topics:
- classic work in areas not treated by any of the existing machine
learning courses
- recent work on the most actively researched topics in machine
learning over the last few years.
|
|
Evaluation will be primarily based on class participation.
Each week a different student will be asked to present a paper from
the literature to the rest of the class.
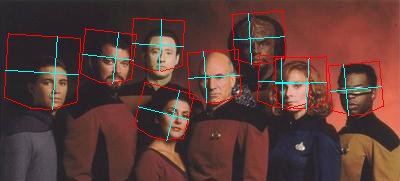 Automatic
Face Detection
Register to the course's mailing list.
Machine Learning Research at NYU |
Please have a look at the research project page of the
Computational and Biological Learning Lab for a few example
of machine learning research at NYU.
There are numerous opportunities for independent studies and even
undergraduate research projects. Contact Prof. LeCun for details.
Code
- Lush: A simple language for quick
implementation of, and experimentation with, numerical algorithms
(for Linux, Mac, and Windows/Cygwin). Many algorithms described in this
course are implemented in the Lush library. Lush is available on the
department's Sun machines that are freely accessible to NYU graduate
students. See Chris Poultney's
notes on installing Lush under Cygwin.
- Torch: A C++ library for machine learning.
Lush is installed on the department's PCs. It will soon be available
on the Sun network as well.
Publications, Journals
Conference Sites
|
 |