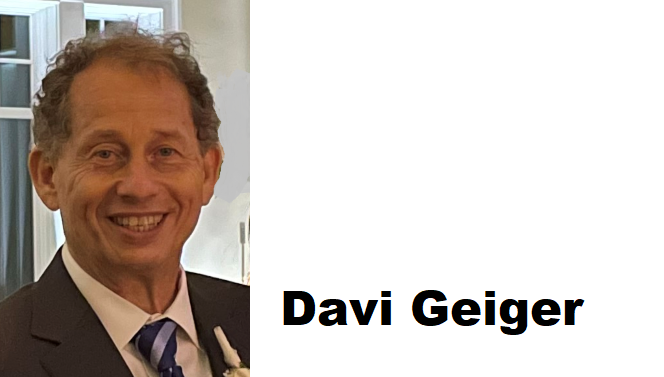
Associate Professor of Computer Science and Neural Science
Courant Institute of Mathematical Sciences
New York University
Co-Founder and AI Leader
Kooick.ai , New York
Nhega.com, New York
Teaching Fall 2024
CSCI-UA.0480-?042. Special Topics: Computer Vision
This course will introduce basic ideas of current Machine Learning methods (Deep Learning with Convolution Layers and with Transformers). The course is a mix of "hands on", with small groups programming/developing projects that integrate the basic ideas above to perform "computer vision tasks" and quizes to cover the knoweldge of the subject. The vision tasks will be in Object Detection, Classification, Segmentation, Depth, and Human Actions.
My critic: Machine learning (ML) gives us today the best tool/method for prediction. However, it is not a causal method and does not reason at the time of execution. If Physics was developed by collecting data of falling objects the predictions for falling objects would be accurate, but one would not learn the causal relation F=ma and F=mg, where g is gravity.
Analgously, ML does not teach us how a machine recognizes a face, but allows us to make a machine that with high accuracy recognizes a face. Moreover reasoning at the time of execution is non-existent. Learning causal relations, in principle, allows us to make more efficient machines and to be able to apply the same causal relations to other aspects of computer vision, not just face recognition. Today, we researchers, have not yet developed a causal theory of computer vision nor a general accepted mechanism for reasoning.
  251 Mercer Street, Room 407
            New York, NY 10011, USA.
 
dg1 @ nyu.edu
 
+1-212-998-3235
Revisiting Quantum Theory:
The paper below presents quantum physics as a Bayesian theory, a statistical theory of knowledge (degree of belief) and it is subjective (to be precisely defined). It suggests, quantum physics is not a causal theory. Also, and specifically, it derives a probability density function in phase space as well as defining interference and entanglement in phase space.
Quantum Knowledge in Phase Sapce
This probability density in phase space is used for the two recently published papers addressing the existence of a time arrow, the trigger for the collapse of the wave function and the creation and anhillation of particles. The paper on Spin phase space does suggest an anisotropic universe.
On Quantum Entropy
Phase Space Spin Entropy
Journal Entropy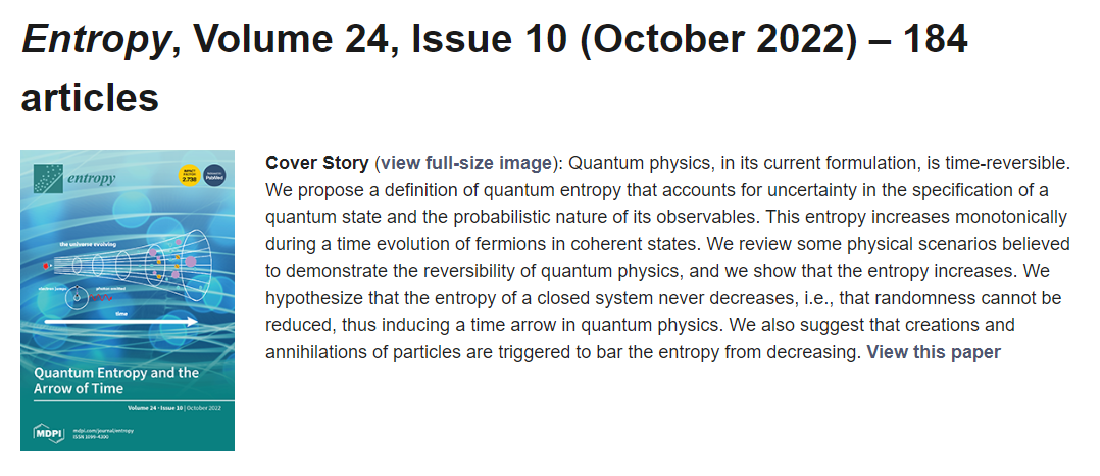